Abstract
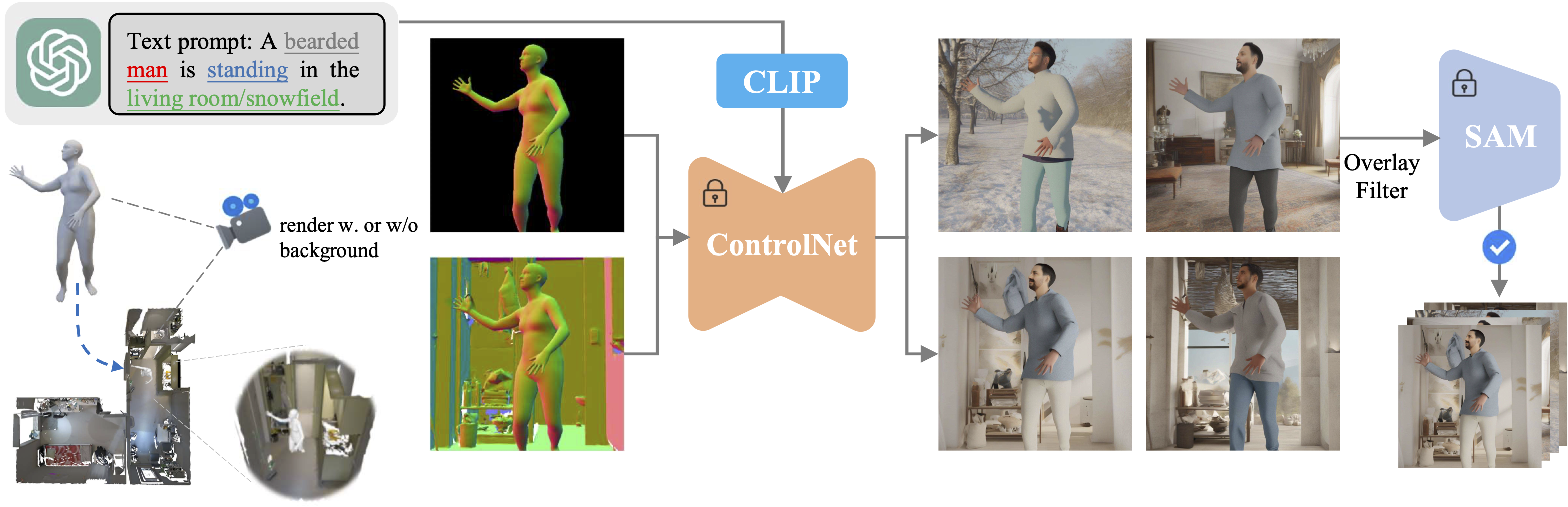
HumanWild from Text and Surface Normal
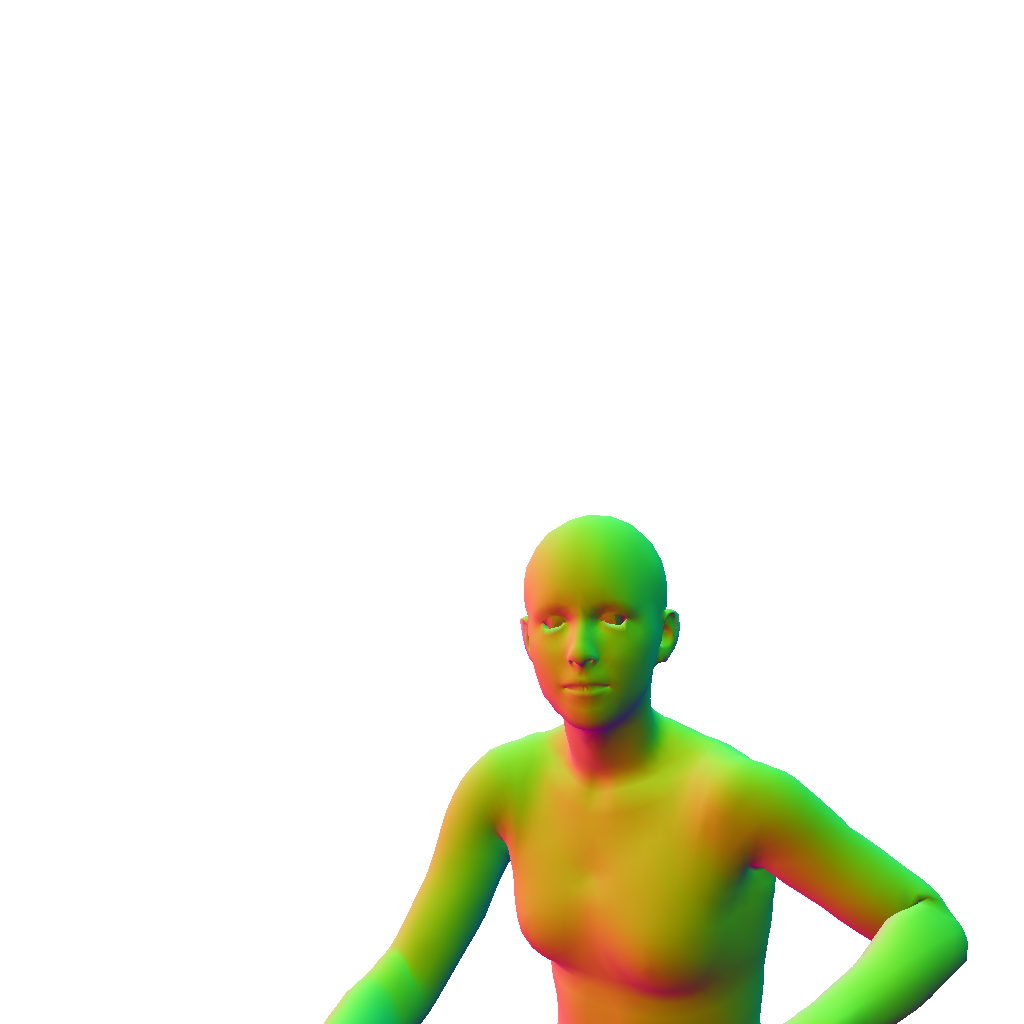
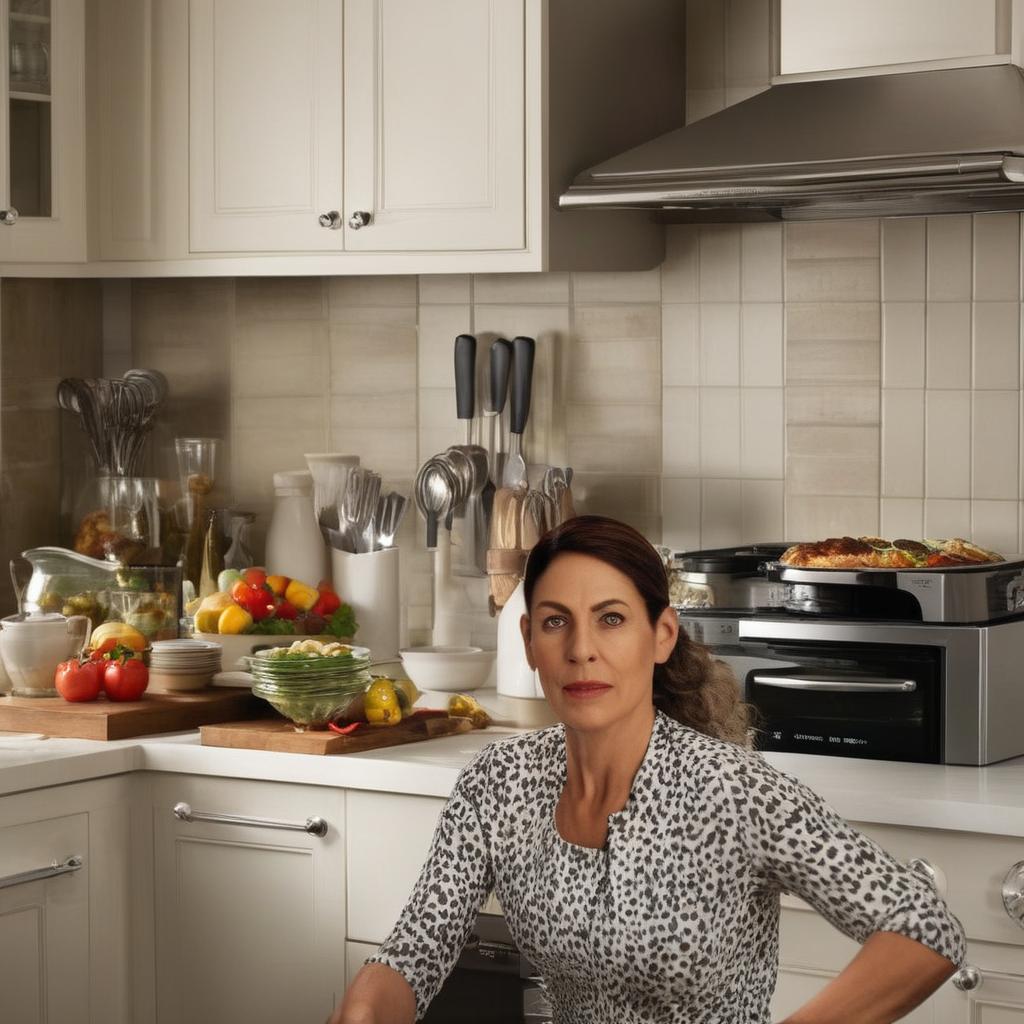
A woman sitting in the kitchen.
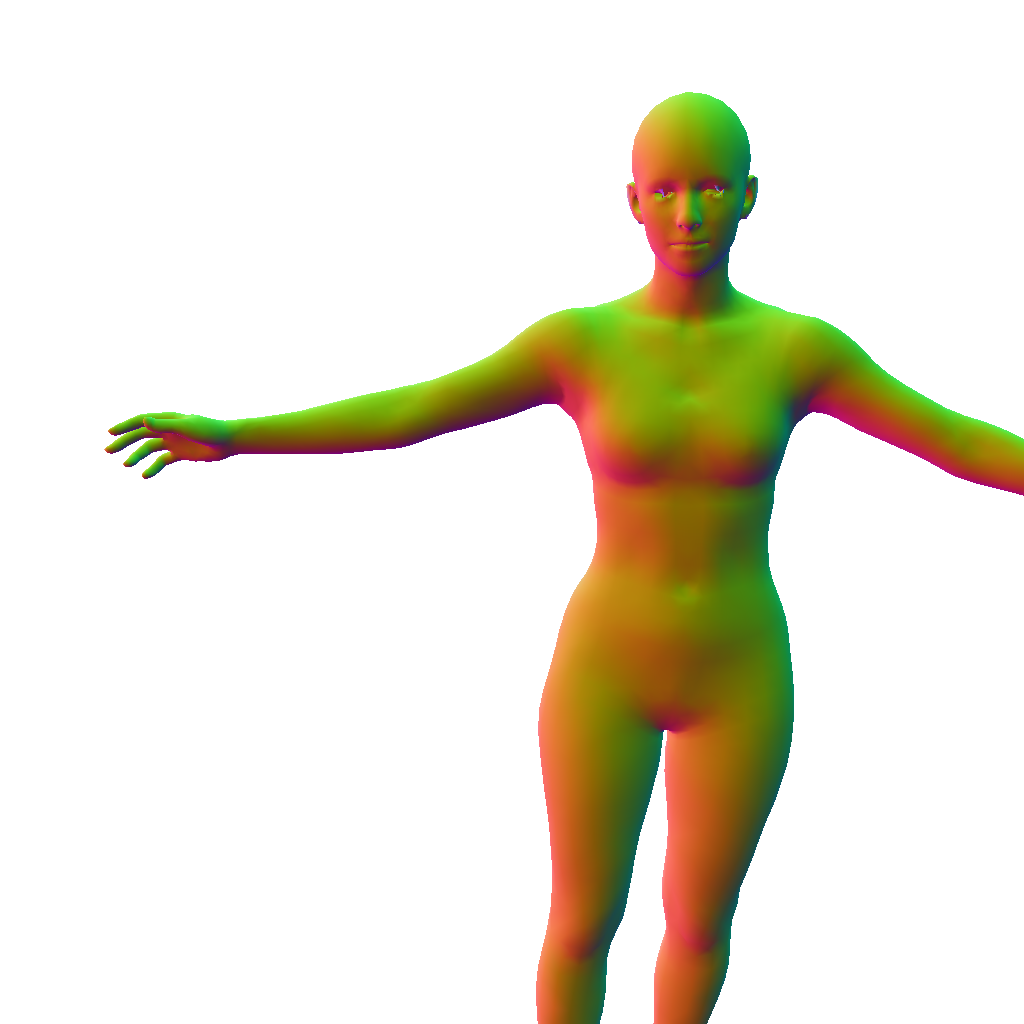
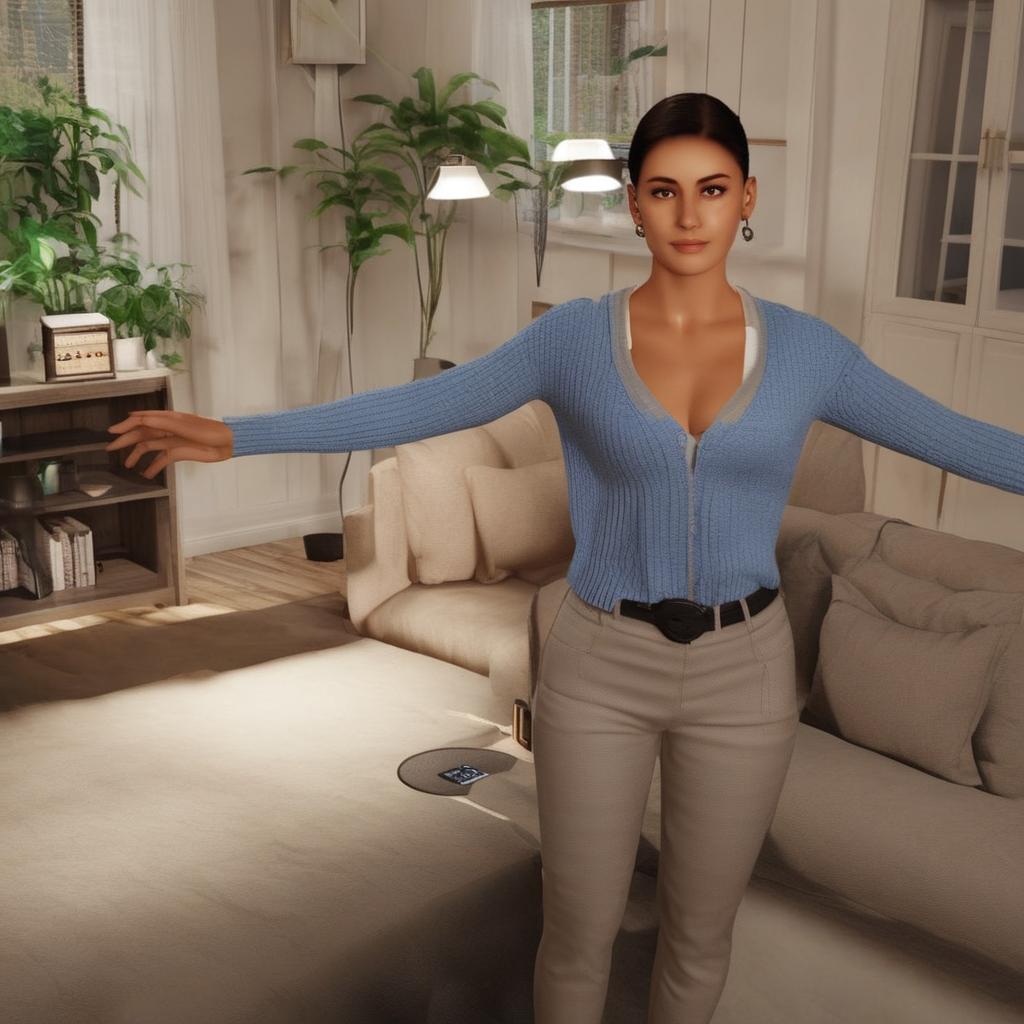
A woman in the dinging room.
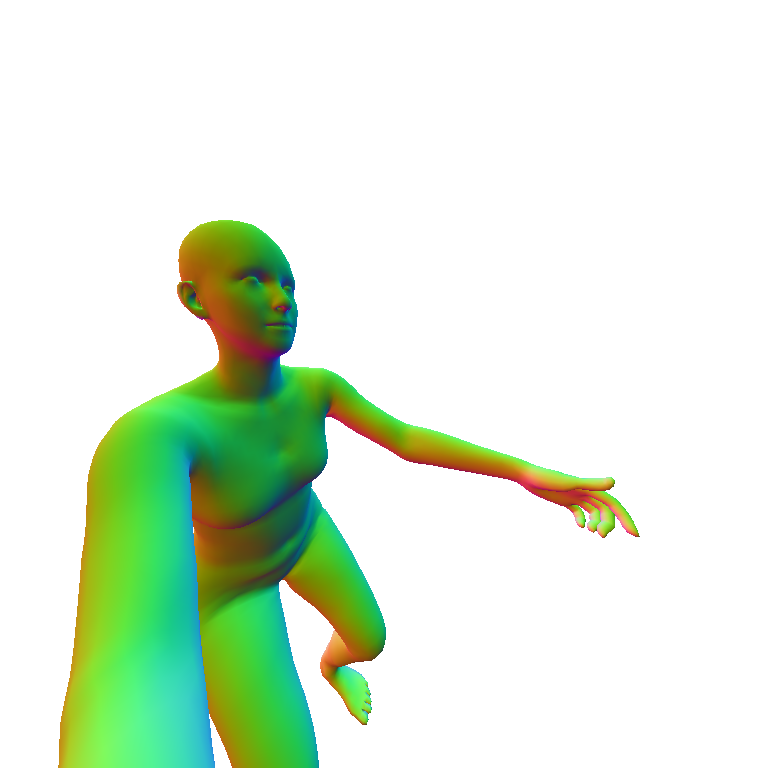
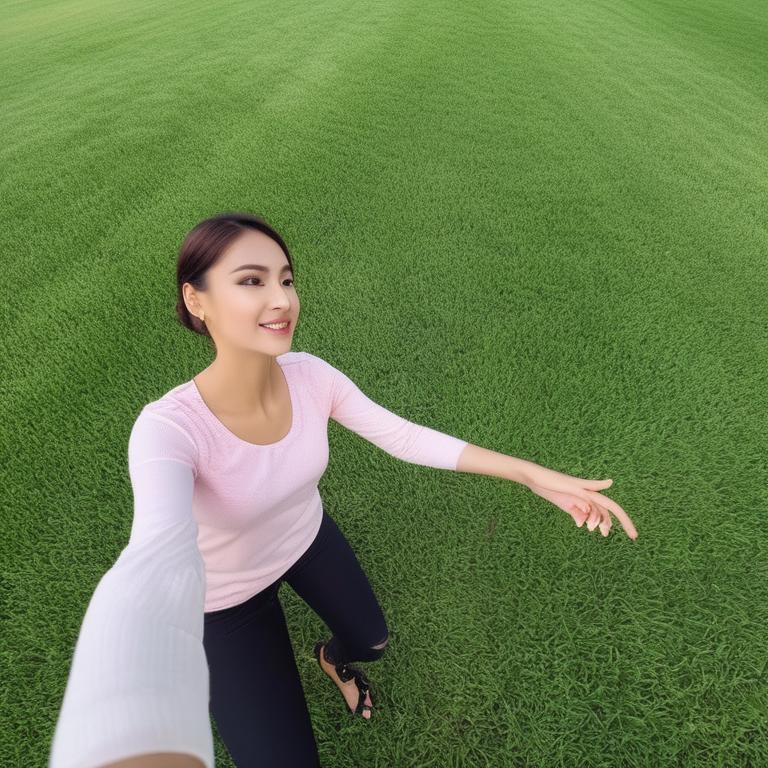
A woman on the grass, distorted camera.
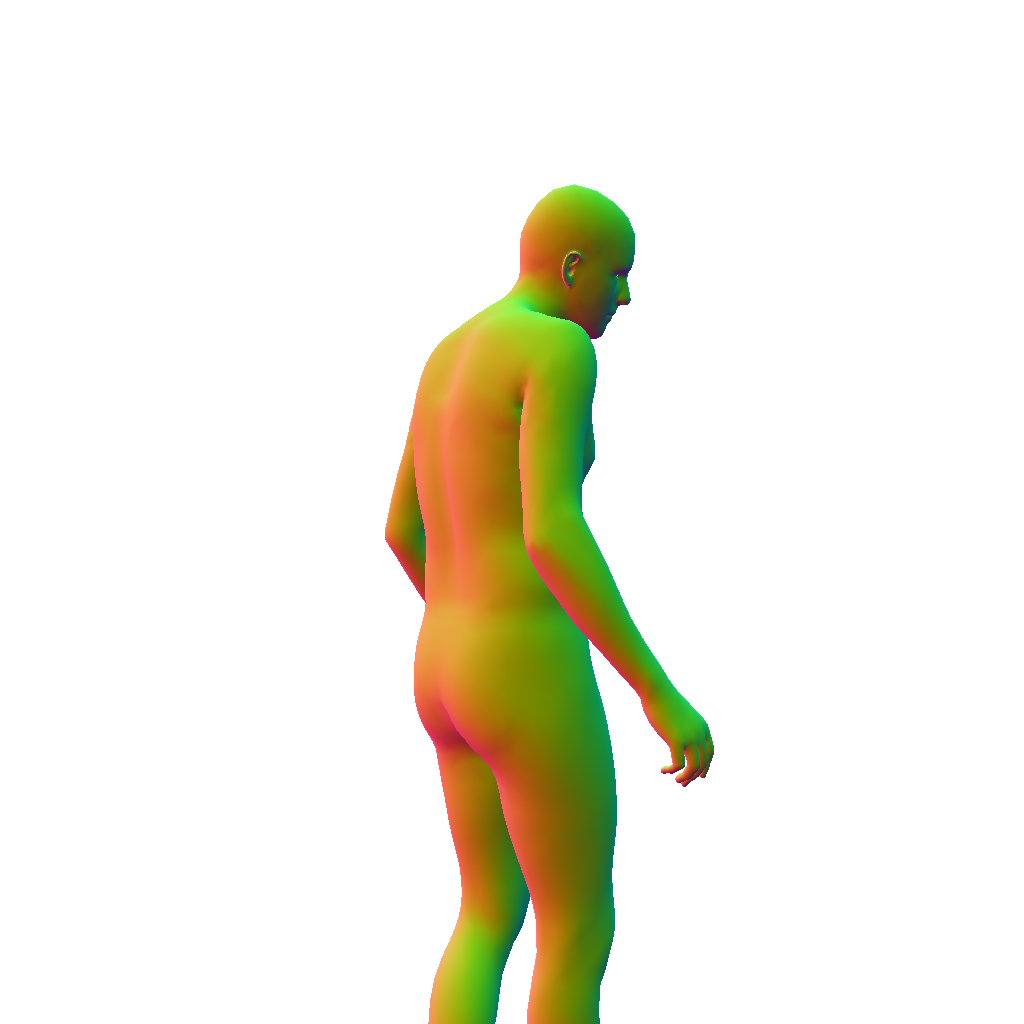
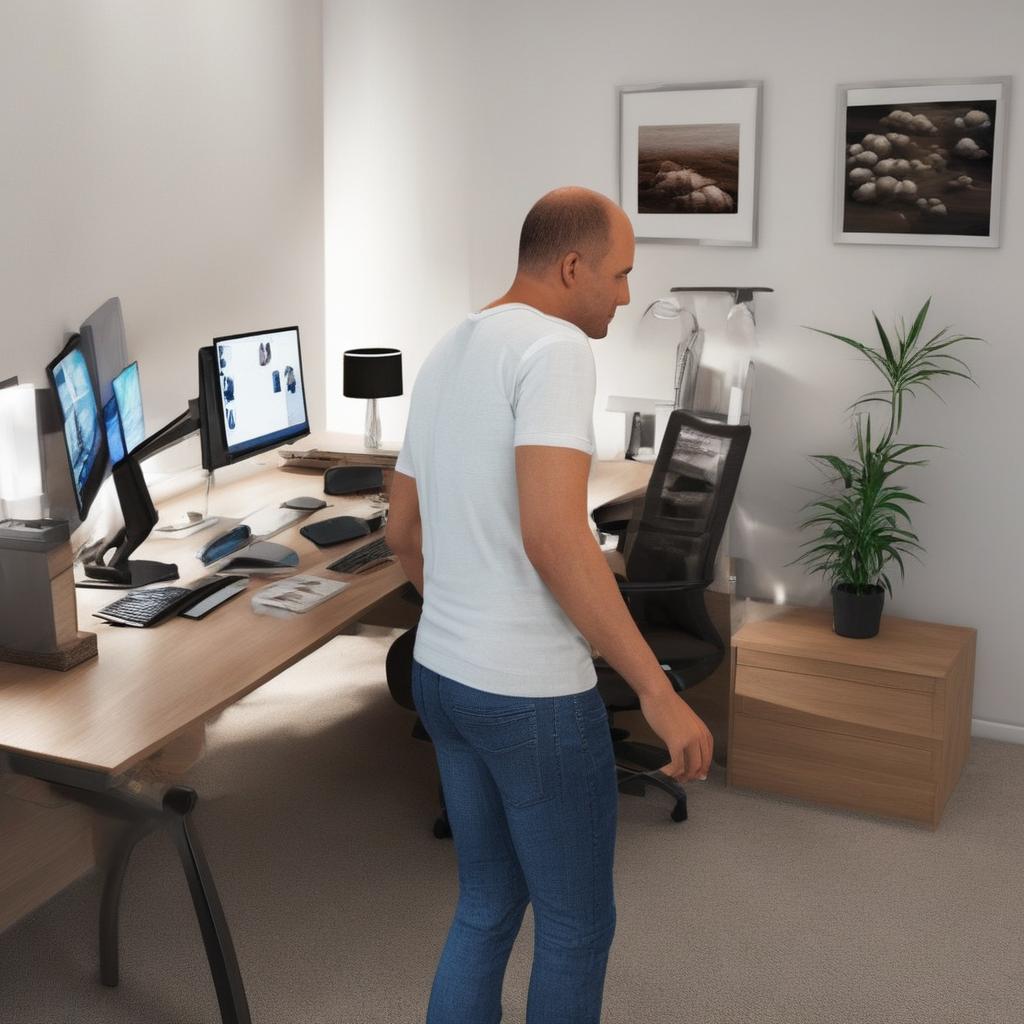
A man in the study.
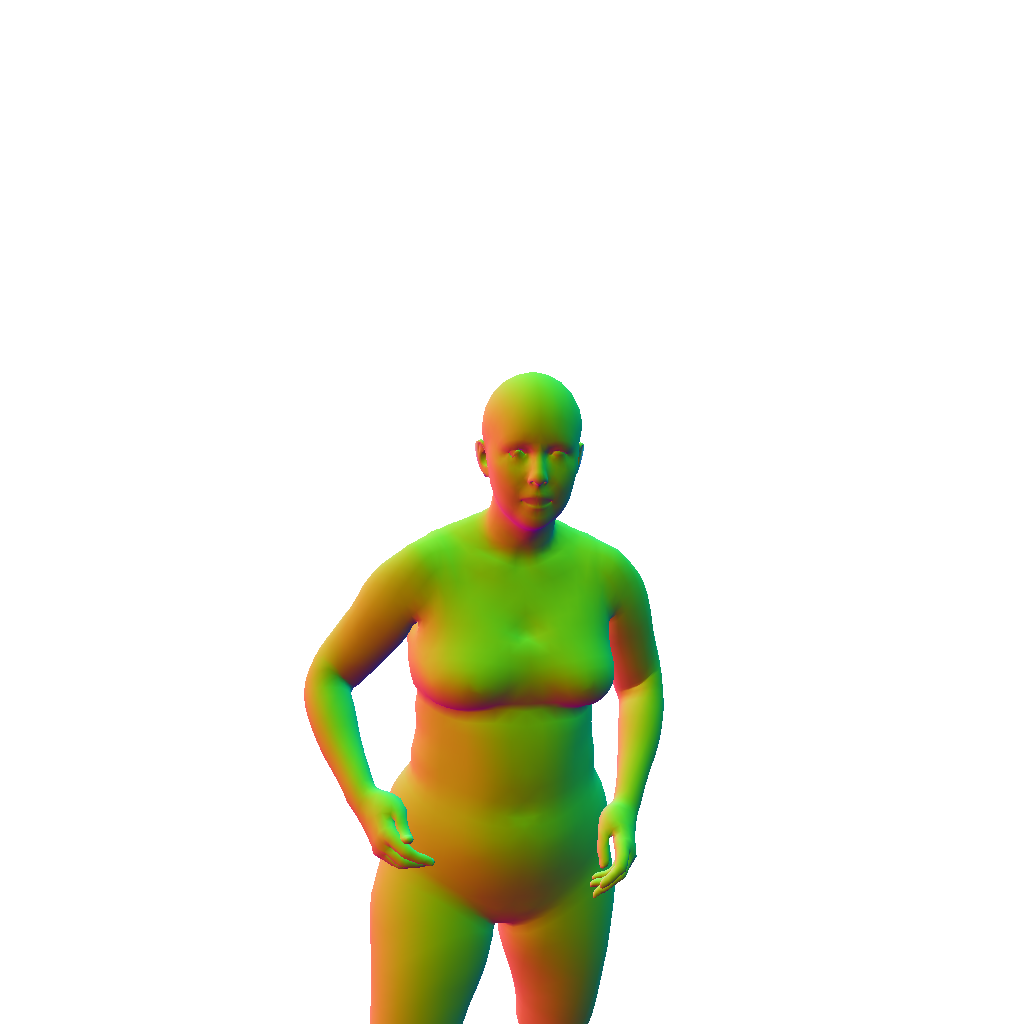
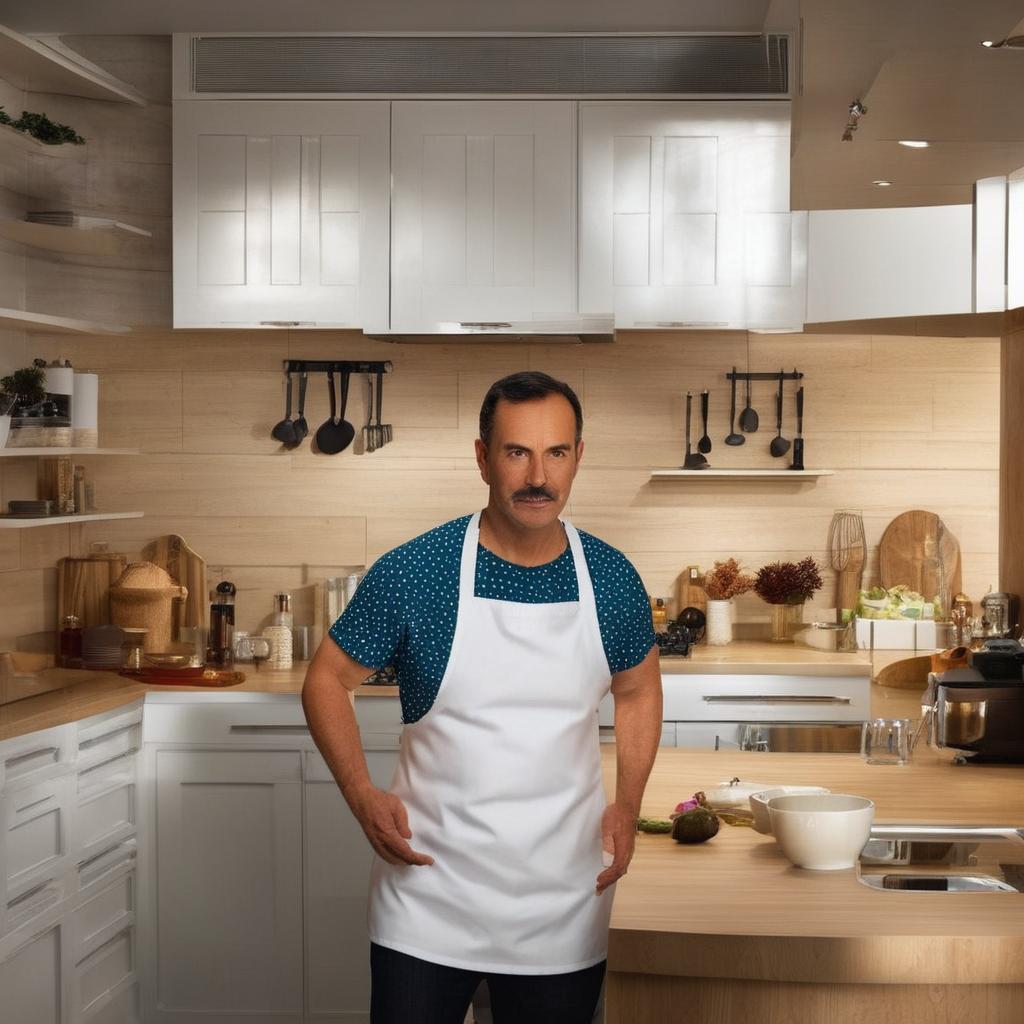
A man in the kitchen.
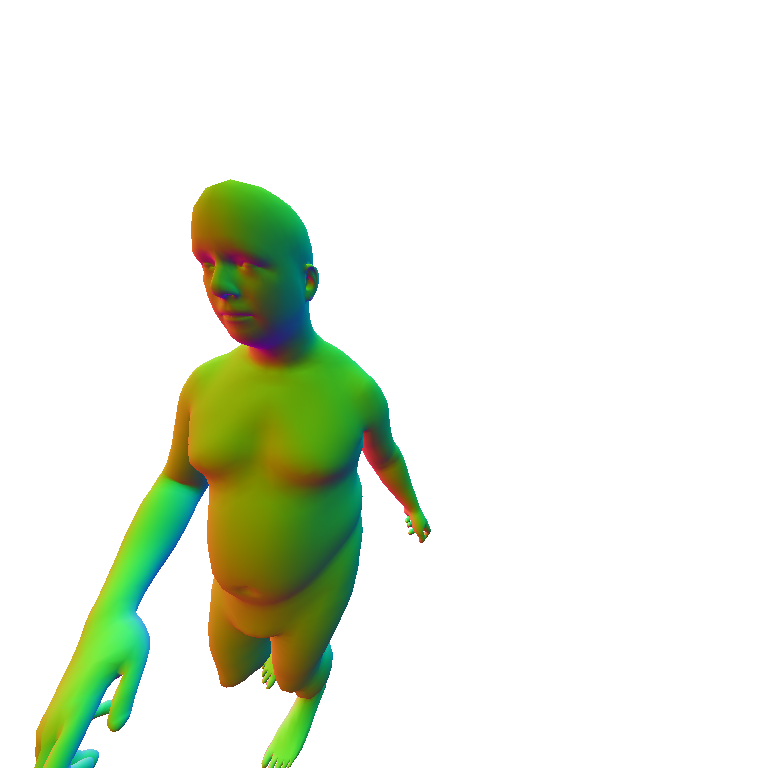
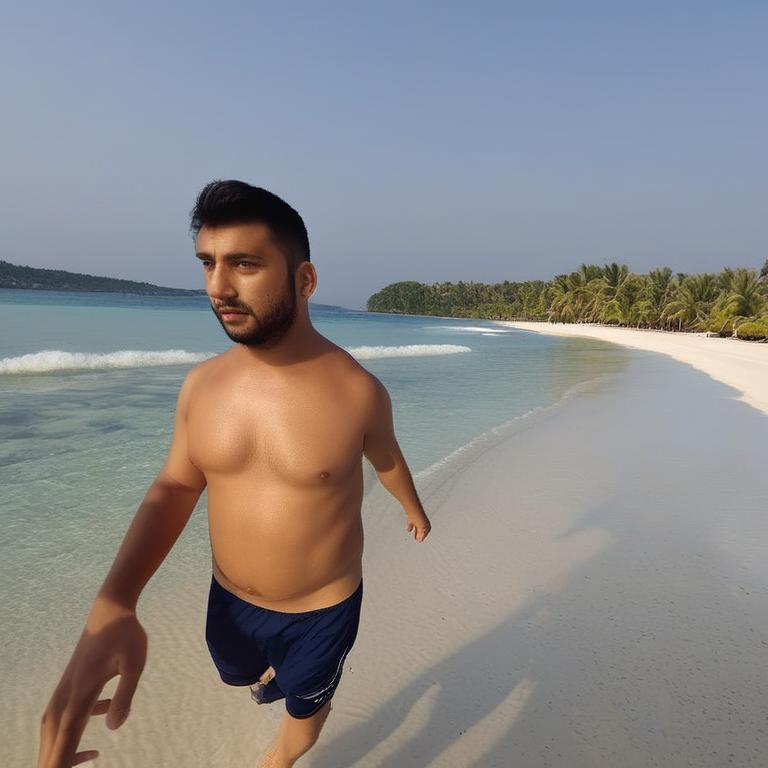
A man walking on the beach, distorted camera.


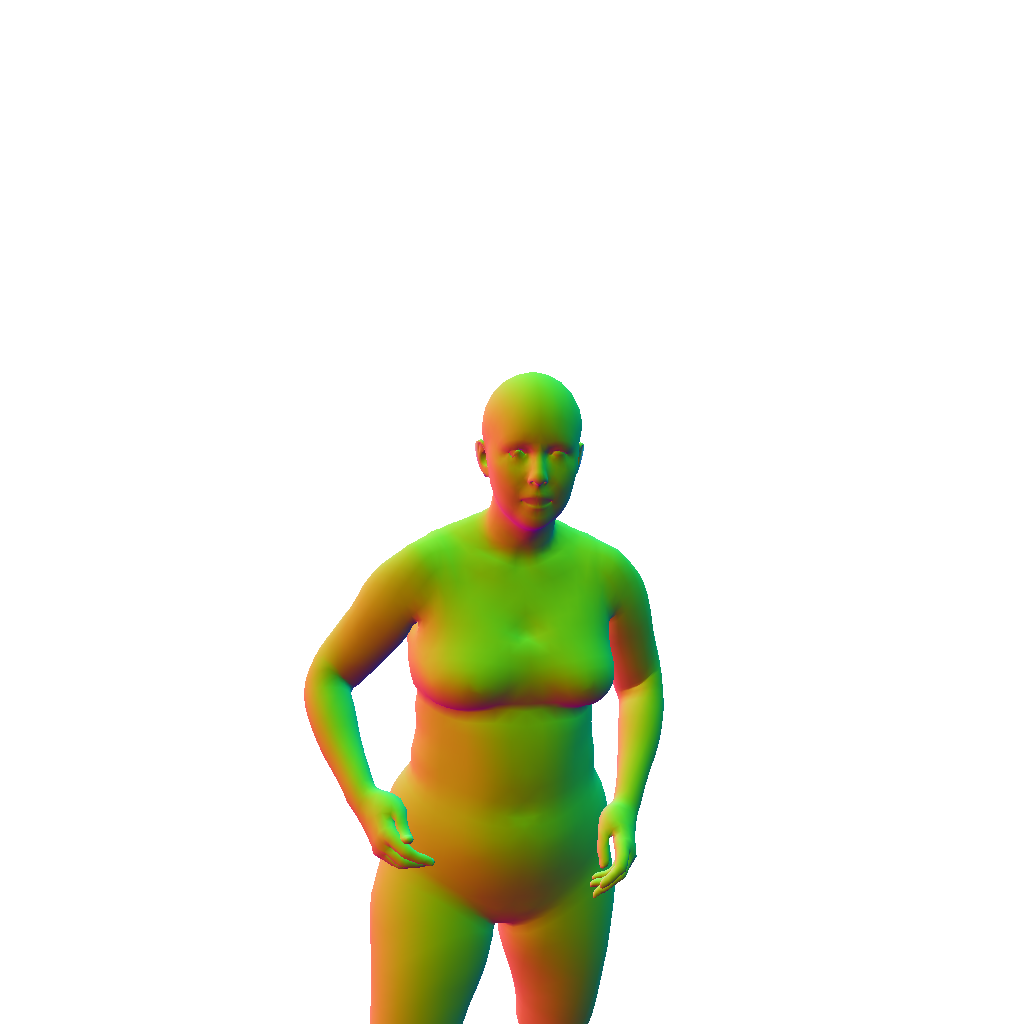

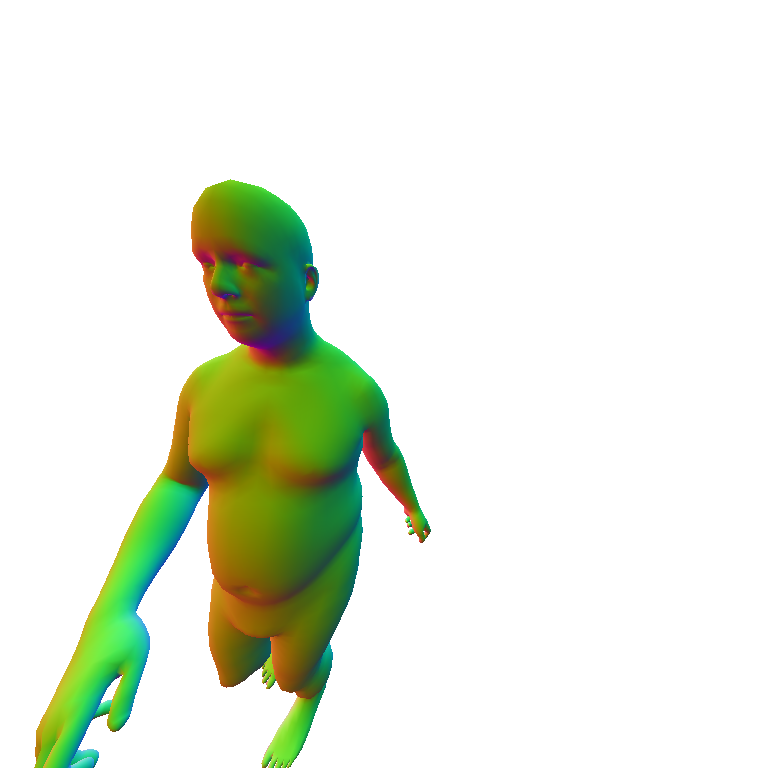
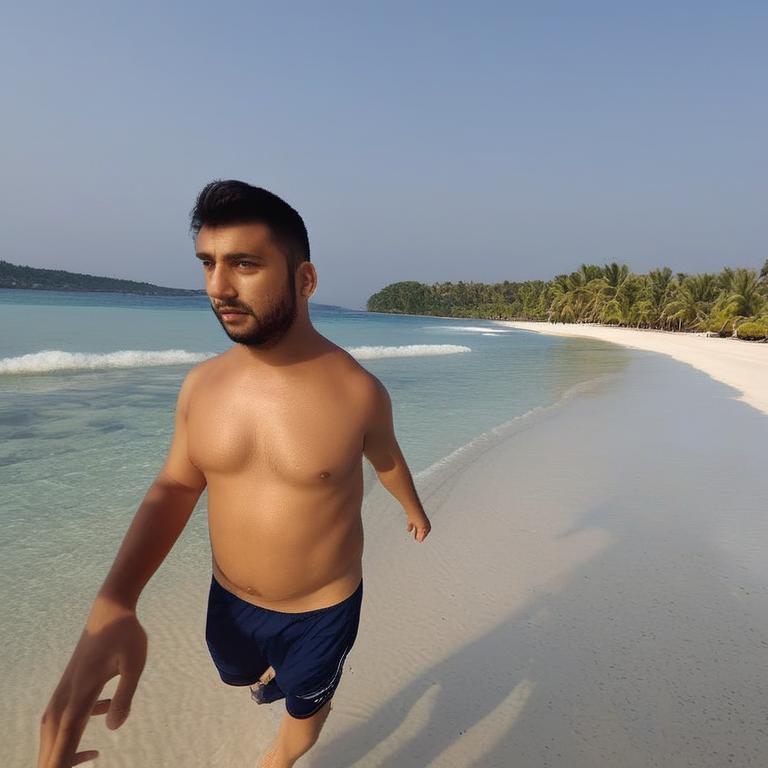
HumanWild with Background Mesh
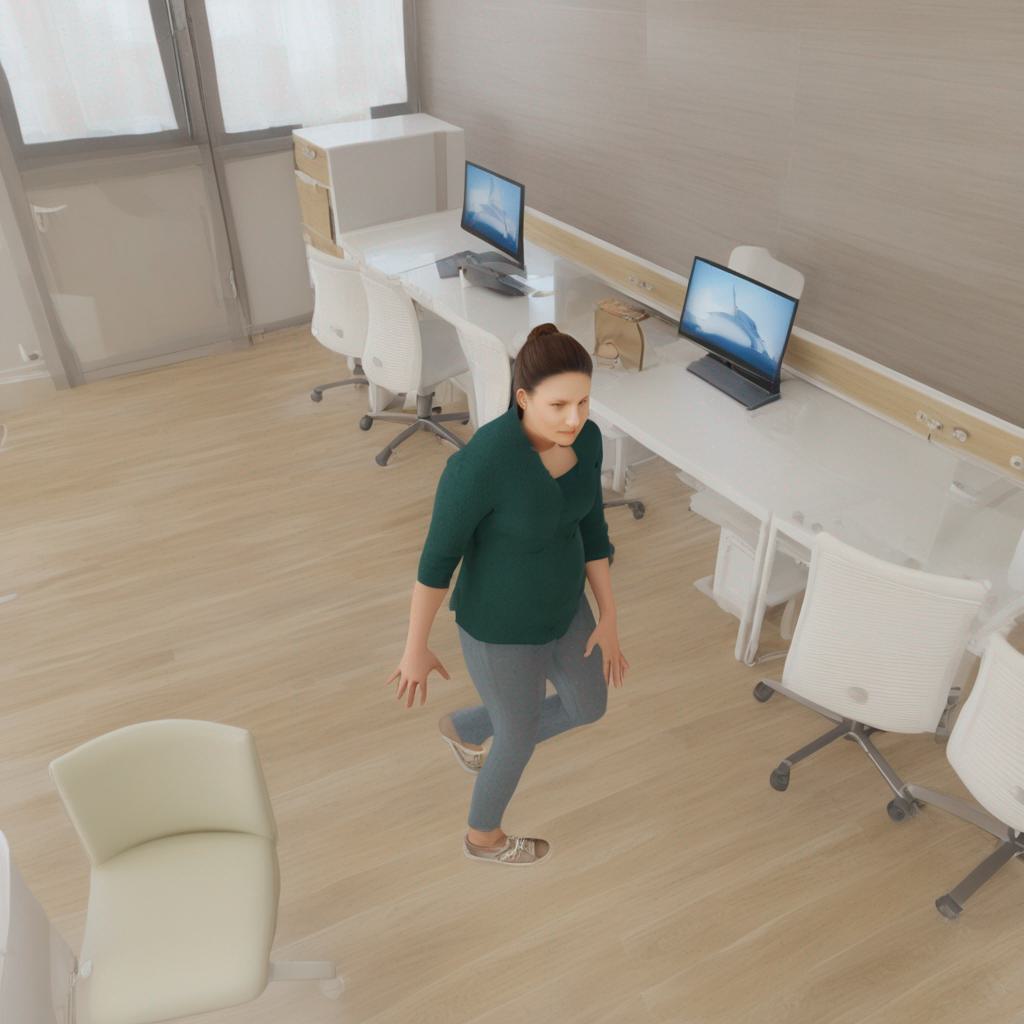
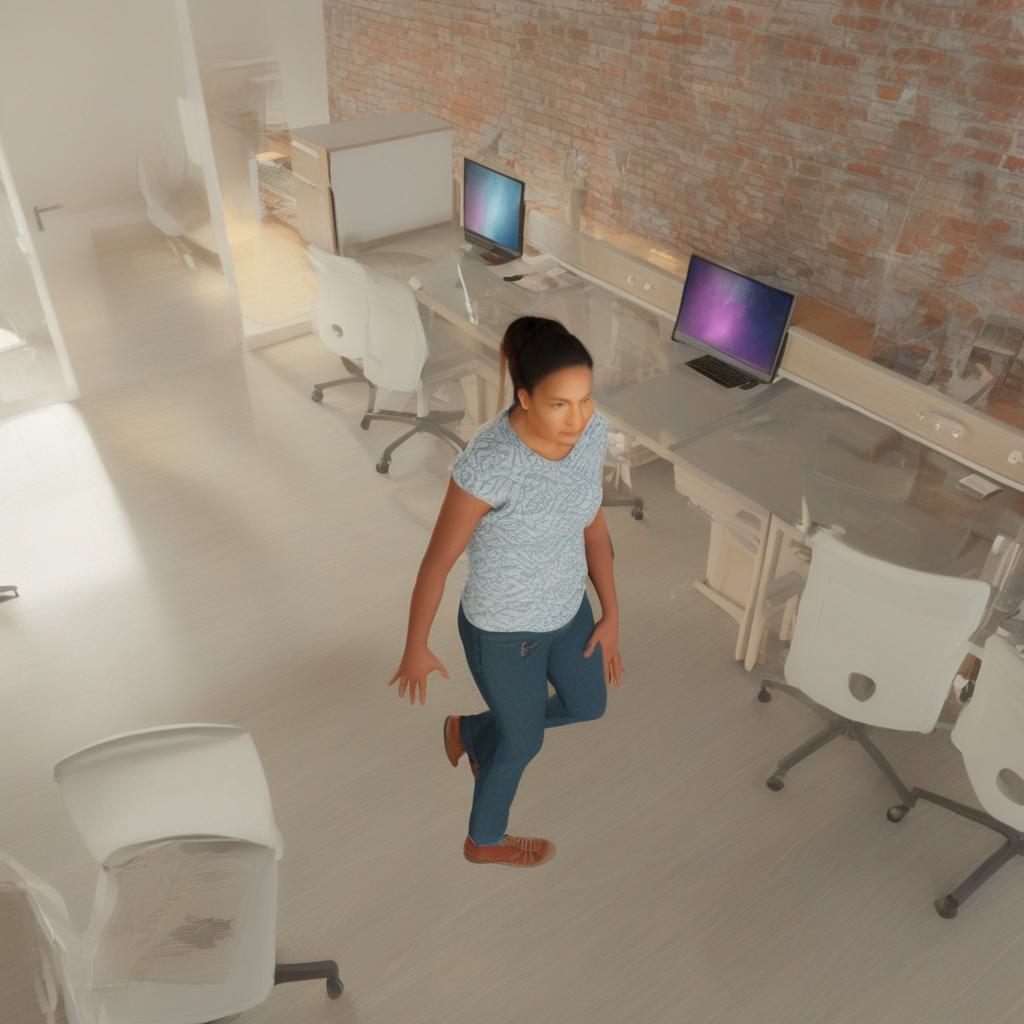
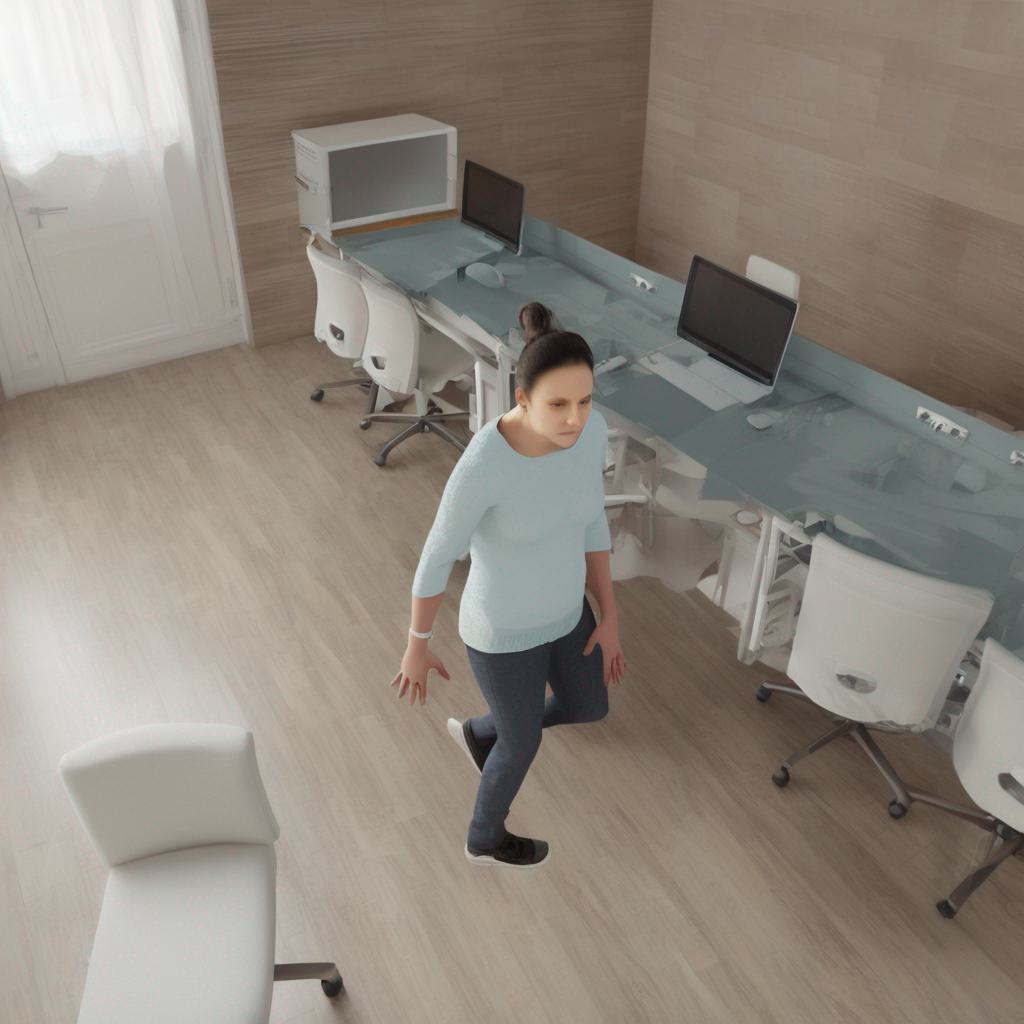
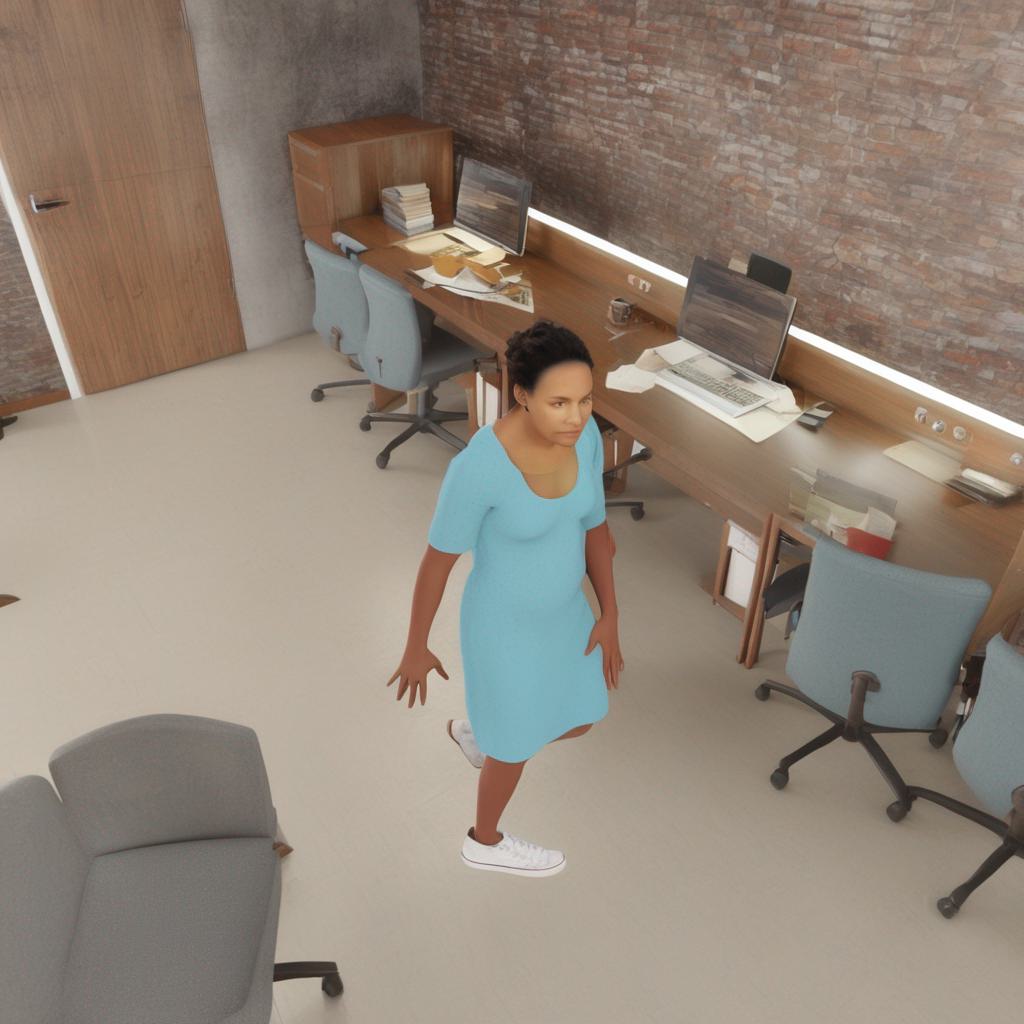
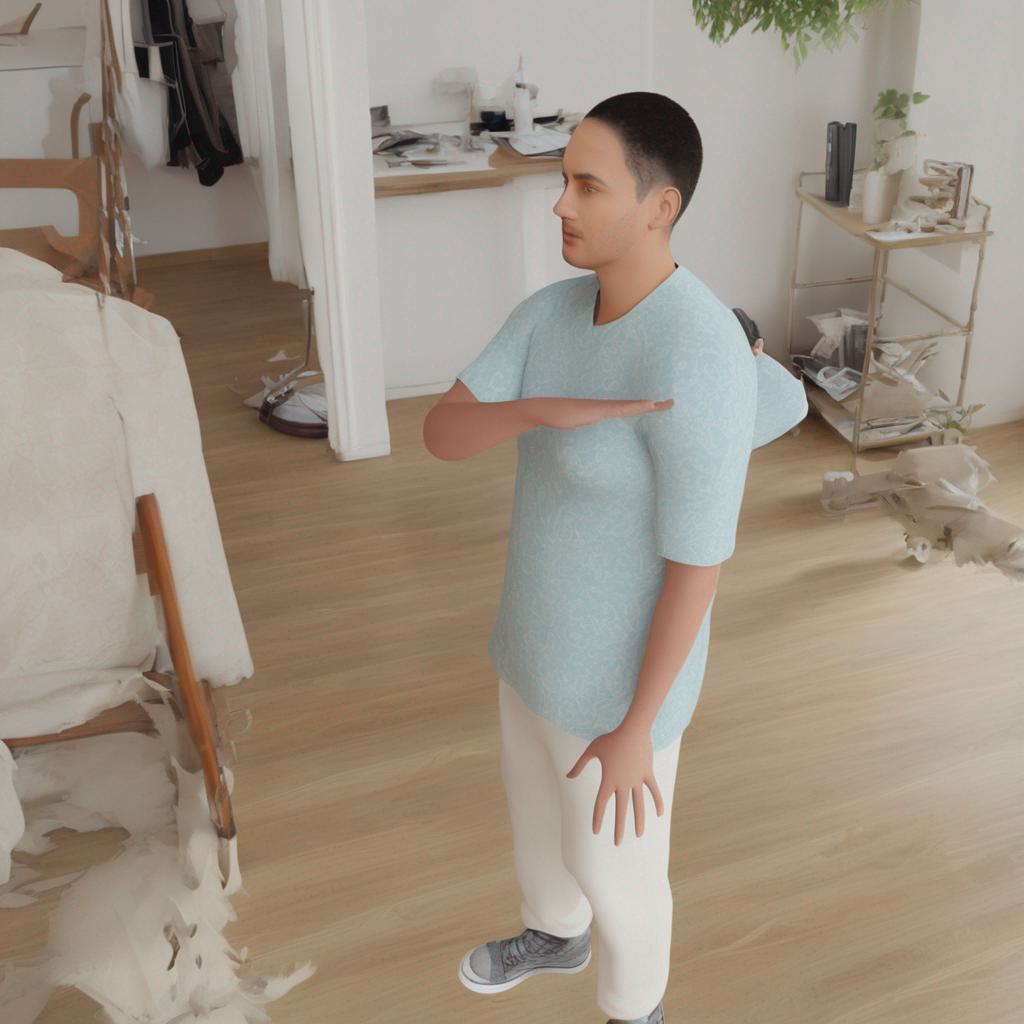
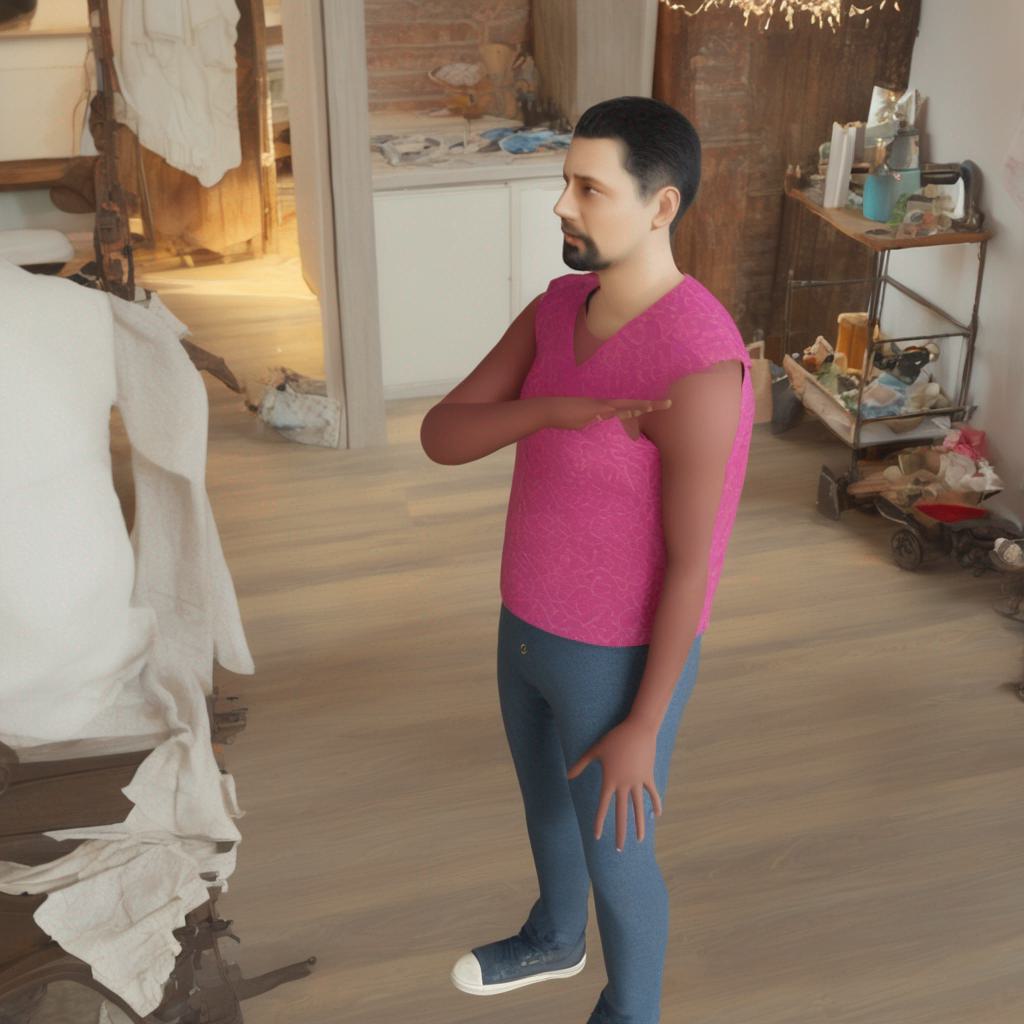
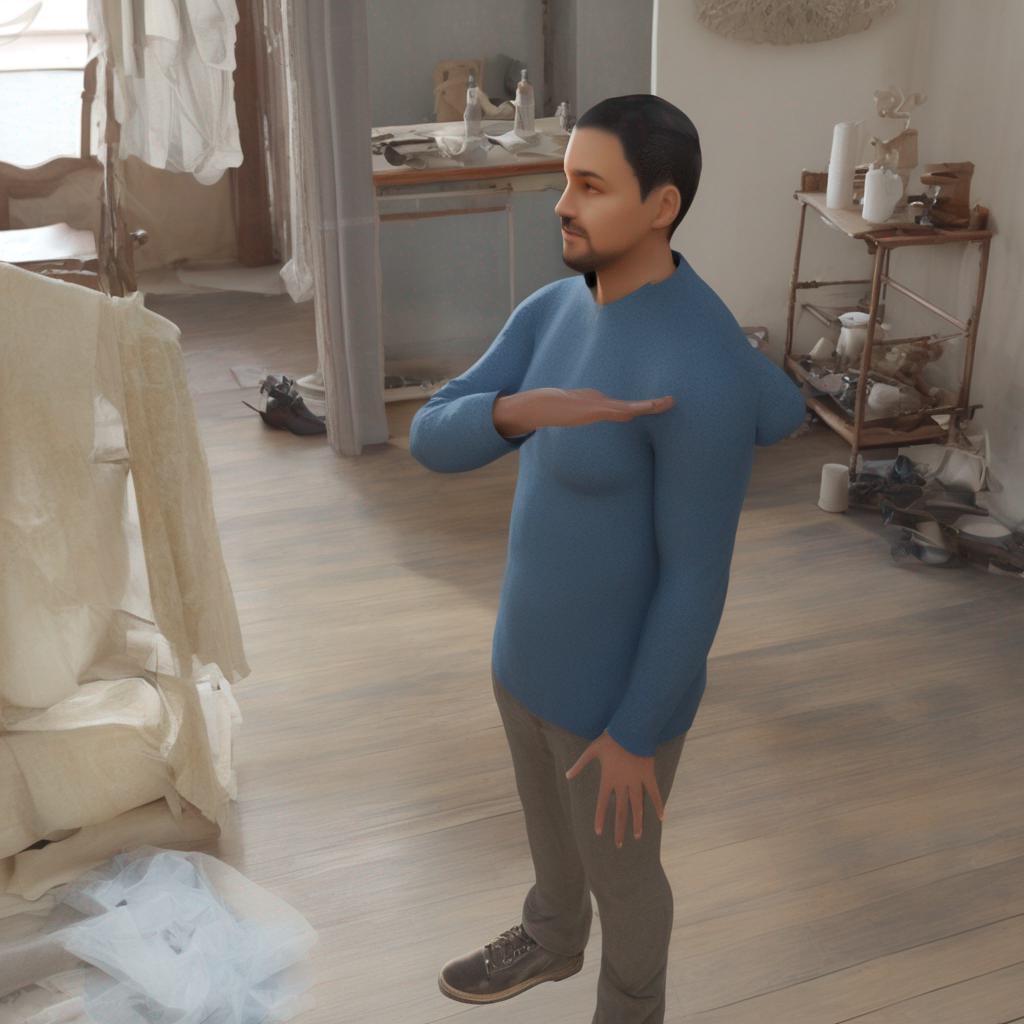
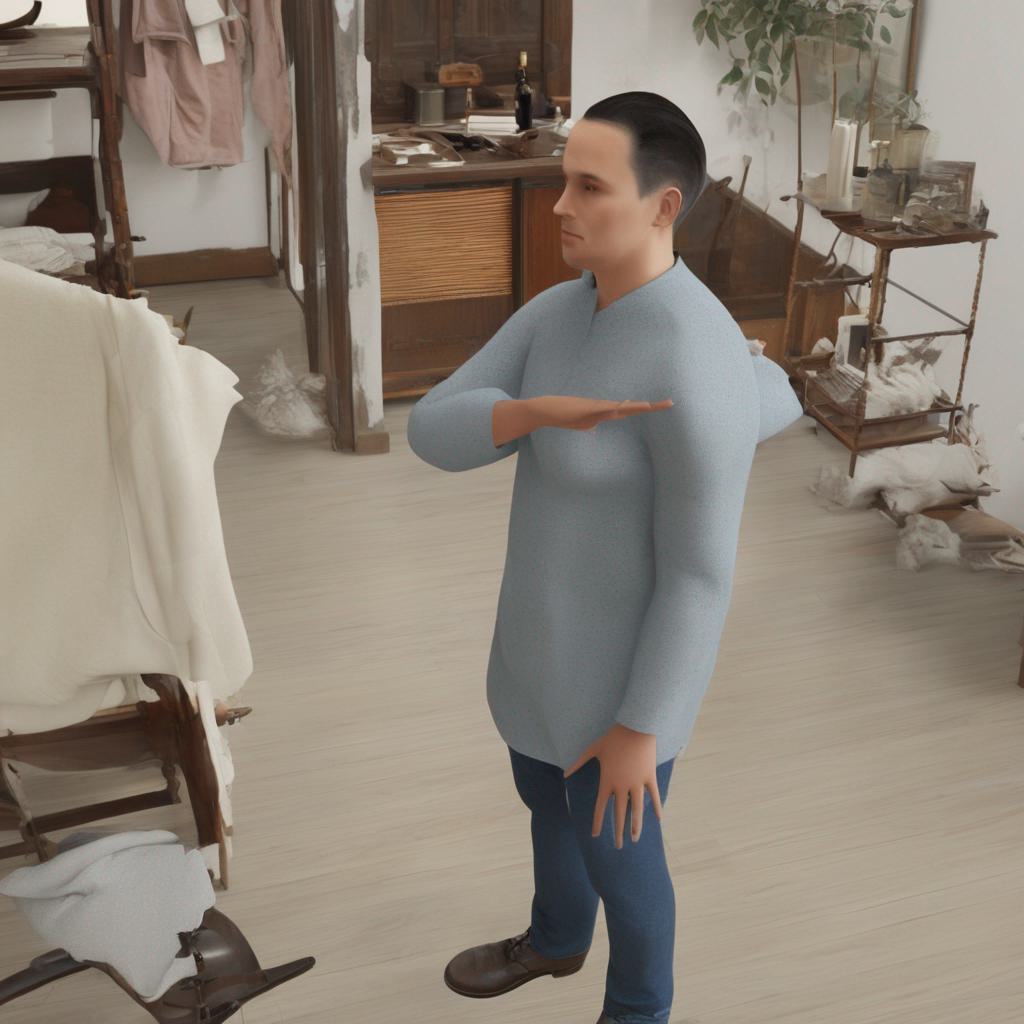
Citation
@article{ge2024humanwild,
title={3D Human Reconstruction in the Wild with Synthetic Data Using Generative Models},
author={Ge, Yongtao and Wang, Wenjia, and Chen, Yongfan, and Chen, Hao and Shen, Chunhua},
journal={arXiv preprint arXiv:2403.11111},
year={2024}
}